Without a doubt, big data and metrics are on the minds of marketers. Yet, few consider applying research rigor to their optimization and measurement plan. Google Media Lab's Tommy Wiles offers four steps to help marketers apply both scientific research and experimentation methods to campaigns.
One of my most hated classes in undergrad was Research Methods. I was drilled on the basics of the scientific method and the difference between causation and correlation, and I was schooled on endless research types (for example, longitudinal vs. cross-sectional studies and pre- vs. post-testing).
Now, as a marketer, I realize just how valuable this required class was. I may not remember organic compounds all that well, or how to take an integral, but my basic knowledge of the scientific method and the benefits of running rigorous experiments has proven extremely useful.
When it comes to measuring marketing campaigns, research methods are often overlooked. However, at Google Media Lab (the in-house team responsible for planning, buying, and placing media for Google), we believe the incorporation of a thorough research process can make the difference between good and bad measurement. With infinite and nearly instantaneous data at your fingertips, robust experimentation can be a determining factor in a campaign's success—and can influence your next one. Here are four steps for applying a research mentality to your measurement.
With infinite and nearly instantaneous data at your fingertips, robust experimentation can be a determining factor in a campaign's success—and can influence your next one.
Four steps to prove marketing impact using experimentation
1. Start with a hypothesis that's grounded in an objective
Smart measurement begins with mapping metrics to real business objectives. To truly understand how to optimize and gain insight from campaigns, however, there needs to be experimentation—and that kicks off with a hypothesis. This gives you a starting point for your testing or investigation.
Your hypothesis should map back to an insight that will help prove the objective of the overall campaign. At Google, we start with the overall business objective before moving on to the marketing and campaign objectives. We aim to focus on a singular objective in each case, so that we have a clear definition of success. Then, we make sure the objectives align and ladder up to each other.
For example, a recent test we ran in an Android campaign rested on this hypothesis: that inclusion of multiple brand elements in the ad units would better support our campaign objective of lifting brand awareness than if we included just one brand element (see graphic in Step 2, below).
When considering a campaign objective, we think about "softer attitudes," such as awareness, education, consideration, and intent; and we break down consumers' actions and behaviors into binaries like trial or purchase and loyalty or usage. We then measure actions or attitudes through online tagging or surveys. More on that in our next step.
2. Devise a robust testing strategy, and don't mistake correlations for causation
Once you've figured out your hypothesis and tied it to the campaign objective, it's time to create a testing plan. Don't settle for observations and correlations. Instead, design experiments and run them to assign causality.
When it comes to experiments, people often think: "I'll try something different and see what happens." They don't create rigorous research methodologies that test a hypothesis and maintain a control group. When Google Media Lab runs experiments, we define control and exposed groups. This allows us to measure incremental action or attitudes and see how these measures shift after exposure.
Returning to our Android example, once we'd identified our hypothesis, we ran tests using three different banner ads and evaluated two variables: the Android logo and the Android character. We broke the campaign into three distinct groups (see below) and measured each group against a control group that did not see any ads. We then measured "unaided awareness," or a person's ability to recognize a brand without being prompted with possible names. We were ultimately able to determine that the presence of both the Android character and logo resulted in higher brand awareness.
Android Case Study: Testing the Logo and Character
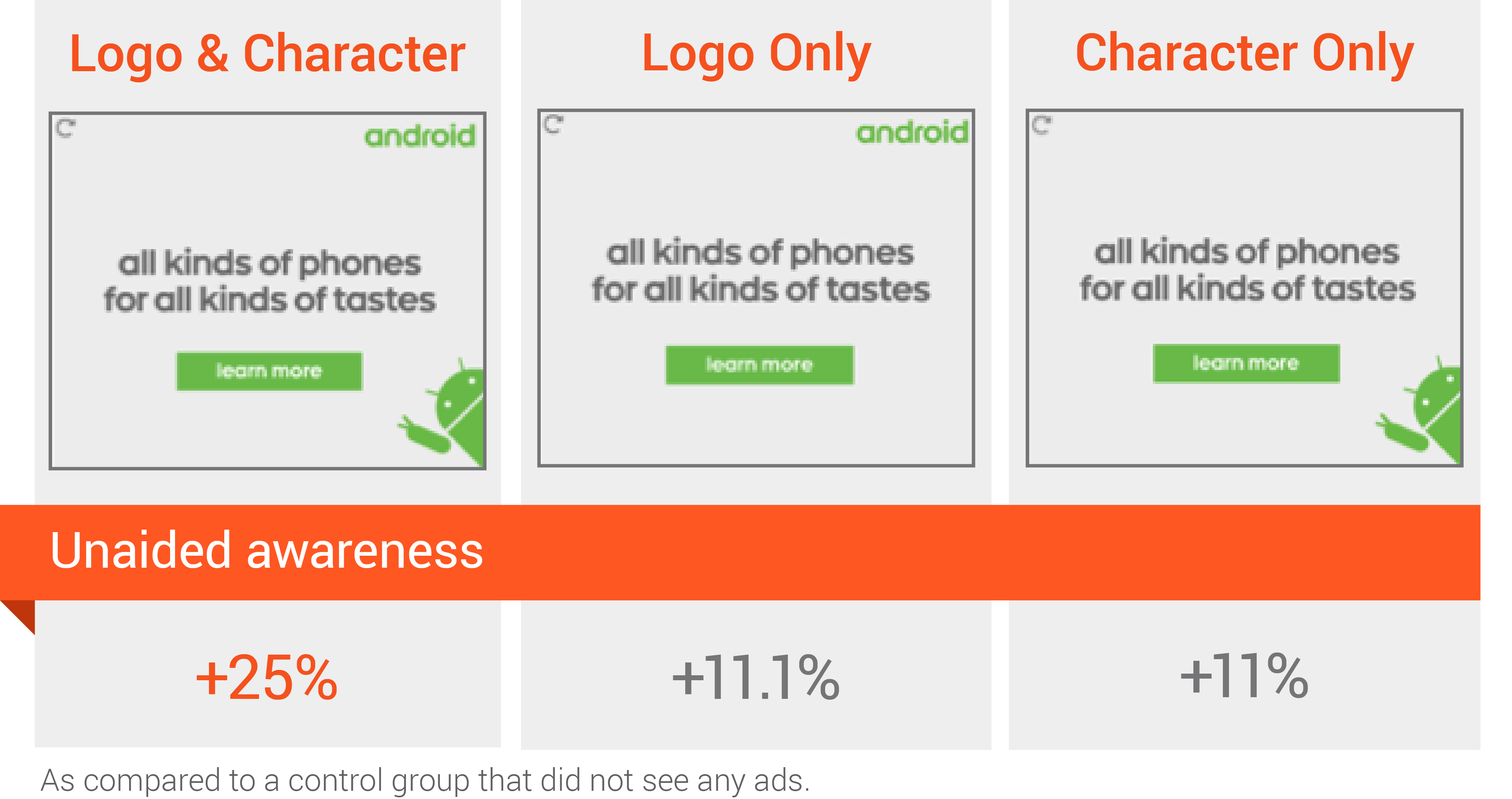
3. Ensure your testing variables are experimentally sound
Researchers often talk about bias in experiments. It's not something well understood by the marketing community, but it happens often. At Google, we avoid bias with the setup that I mentioned above: control vs. exposed groups. We then randomize these groups and measure them simultaneously. This prevents time bias (either pre- or post-) and audience bias from affecting our experiments.
In the experiment for the Android campaign, we set up three groups, each with a control group, for a total of six "cells." We then measured the awareness lift that occurred in each of the groups that were exposed. We also limited the number of variables that we changed when testing something. By controlling for these factors, we could assign causality. Then, we felt confident in the insights we generated and the changes we could make to our next campaigns.
4. Choose robust measurement tools, and deploy them systematically
Ultimately, we know that the power of data comes in being able to slice and dice it in many different ways. That's where your measurement tools come in. We work with tools, such as DoubleClick and Google Analytics Premium, to organize our data signals (for example, location, time of day, past behavior and interests, and website usage).
These tools help us use the right data to reach the right audiences with our campaigns. Imagine the benefit of having the data and insights gained from previous campaigns at hand for your next programmatic buy (the focus of the first piece in our "Inside Google Marketing" series).
We can then extend these insights and tests to other brands within our portfolio. This allows us to gather best practices across brands and campaigns. At the end of the day, we can assess and compare dollars being spent across multiple campaigns, and report up to our stakeholders with confidence.
While Steps 1-3 above will work for a single campaign, ideally you want to systematize measurement by using the same tools and methods to see insights across all campaigns. In doing so, you can make powerful cross-campaign, cross-publisher comparisons, and gain insights to break through today’s vast multi-channel ecosystem.
Smart measurement is rooted in research
These days, there's a lot of talk about big data and the power of marketing science. Smart marketers approach measurement with a research mentality. They understand the need to root their measurement in objectives to ensure that their techniques draw experimentally sound insights. By standardizing tools and systematically deploying them across campaigns, they can easily compare efforts and results. Most importantly, they gain an understanding of what worked and what didn't.