Gathering the right insights to deliver helpful customer experiences starts with ensuring your first-party data is streamlined, consolidated, and privacy-centric. Here, Christian Johansson, head of marketing and digital at RAMS, and Rachida Murray, national head of digital and technology at the financial institution’s agency, Spark Foundry — part of Publicis Media — share how they revamped their data strategy to better engage prospective customers online.
While there’s no place like home, we’ve seen first-hand at RAMS that navigating the mortgage market is understandably challenging for many Aussies. That’s why since 1995, we’ve been offering tailored services and sharing online tools such as home loan calculators with homeowners who need a helping hand.
But as Aussies’ financial priorities have shifted along with banking regulations, we realised we could do more to connect people with the services they need. Here’s how our teams at RAMS and Spark Foundry took a leap and used automation to fuel our campaigns with a smarter, data-driven lead scoring model.
Getting a comprehensive understanding of our customers
To figure out what was most relevant to Aussies as they researched home loan options, we wanted to get a better idea of how they interacted with us from their first search to final application. Connecting the dots throughout our customers’ journeys was challenging because RAMS’ first-party data comes from siloed sources — from our franchisees to our website. So, the first step was stitching together a holistic dataset in Google Analytics that aligned with our privacy-centric policies.
By consolidating all our data, we were able to surface insights about which services were most helpful to our existing customers. Then, we developed a new lead scoring model in Google Tag Manager with our privacy-forward data. Because we defined our lead scores by cross-referencing them with aggregate attributes that were similar to those of RAMS’ customers, we were able to ensure that all personal identifiable information (PII) remained confidential.
Because we defined our lead scores by cross-referencing them with aggregate attributes that were similar to those of RAMS’ customers, we were able to ensure that all personal identifiable information (PII) remained confidential.
Harnessing the power of machine learning
Once we created aggregated lead scores with Tag Manager, we stored them in Analytics to use them as value-based signals for our automated target return on ad spend bidding. While our lead scores didn’t capture any PII, we were still able to use them to serve Search ads to Aussies whose applications closely mirrored those of RAMS’ existing customers. That meant we could focus our campaigns on reaching people most likely to need our services.
After reworking our strategy, we drove up applications from paid search by 168% and lifted our lead-to-application rate by 56% year over year (YOY) between July and September 2020.
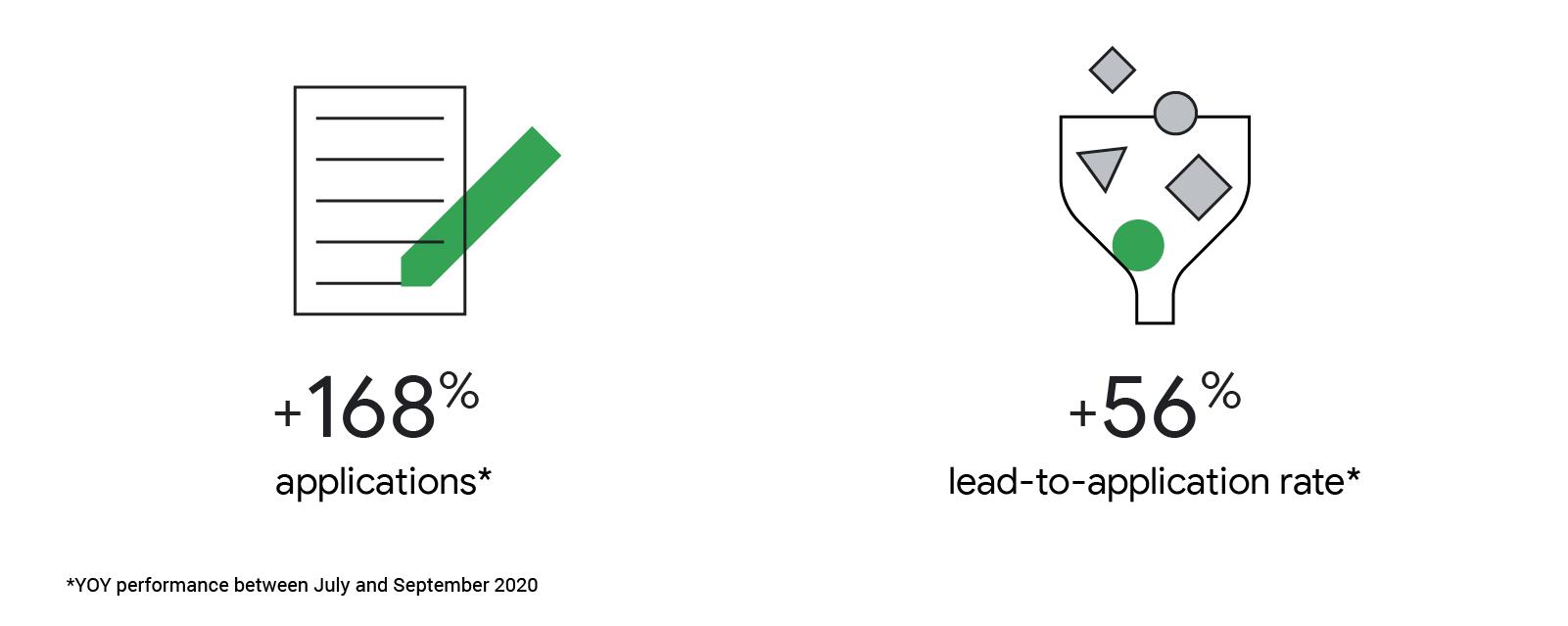
Even more, we increased lead volume by 72% while decreasing cost per lead by 47% and overall marketing spend by 10%. And we’ve continued to build on our success by experimenting with Maximise conversion value bidding, which automatically adjusts our bids to prospective homeowners’ shifting search behaviours.
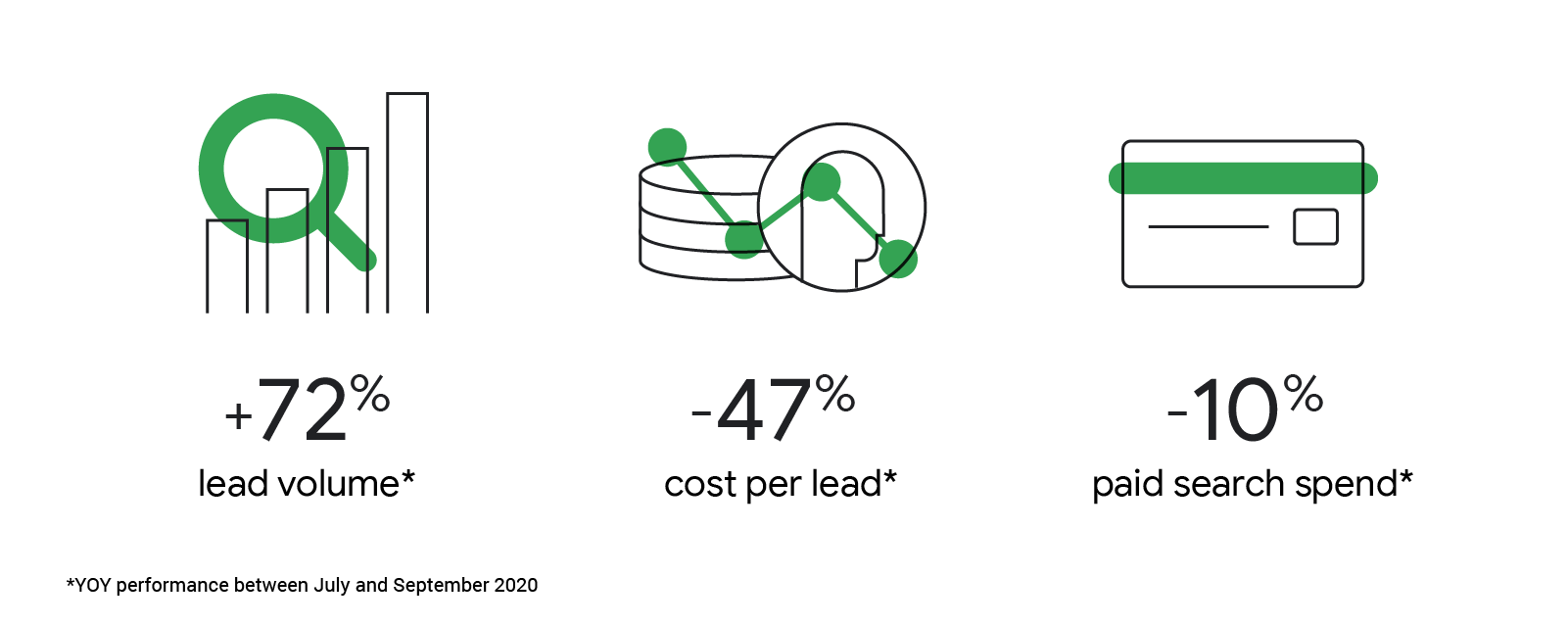
By using automation to reach more potential customers, we learned how to use our budget more strategically to create better, more helpful experiences in a privacy-forward way. Working with less data is becoming the new normal as people grow more protective of their personal information, so it was promising to see we could drive greater performance and relevance without using PII.
Working with less data is becoming the new normal ... it was promising to see we could drive greater performance and relevance without using PII.
As we continue to step up our services for prospective homeowners, we’ll keep in mind the biggest lesson we’ve learned: We don’t need to overthink what it means to be data-driven. Simply focusing on the insights that matter most to our goals is more than enough to pave the way for long-term growth.