A new white paper shows how India’s Ministry of Civil Aviation sought Google’s support to parse econometric, dynamic data in real time to help pick sites for future airports ― and how "location-casting" can benefit decision-makers in almost any industry.
Setting out a plan for facility locations is a classic challenge for organizations of all shapes and sizes. This is the case for companies around the world — from retailers to governments and corner stores to department stores — but especially in India. Because healthy returns and high efficiency sit at the core of both public and private entities, leaders are continuously reaching for the optimal point where projected demand meets ROI.
Traditionally, demographic statistics and survey-based reports have been computed by standard algorithms. Now, with Google’s "location-casting," companies can use artificial intelligence and machine learning to sift through huge amounts of anonymized data and search queries to suggest a set of locations, that simultaneously takes into consideration factors like consumer demand, effective costs, profits, and future sites.
Success story: locating India’s next set of airports
India's Ministry of Civil Aviation was one of the first organizations to use location-casting to address the country’s complex network of airports. Its goal was to understand not just where passenger demand was the greatest, but also which combination of airport sites would meet that demand most efficiently and with the greatest ROI.
When its ambitious program of Ude Desh ka Aam Naagrik ("A Common Man Can Fly") began in 2016, India had only 75 airports — considered low for a vast country of more than a billion people (compared to over 200 in China and 5,000 in the United States). The ministry’s research revealed two ideas that served as the foundation for its year-long query:
- Domestic airlines carried nearly 117 million fliers in 2017 alone (a 17% increase from the previous year)
- 97% of India's population had never set foot on an airplane.1
Given India's fast-growing population and thriving economy, it became evident that new fliers would soon overwhelm the country's aviation infrastructure. More airports were needed, and fast. But the ministry still wondered: which network of city and regional airports would maximize convenience for the most people without creating redundancies? To find a solution, the ministry needed to answer a host of complex questions:
- Where is demand the greatest? Which areas have both broad interest in travel and the broad affluence needed to afford that travel? For example, the presence of increasingly heterogeneous industries is a good indicator of an area’s affluence in that it demonstrates its ability to sustain a diverse ecosystem.
- What is the optimal network of airports? What network of locations would get people where they want to go while reducing travel overhead and working well with the country's widespread network of highways and railway lines? Take, for example, Jalandhar and Ludhiana: although they’re just 60 kilometers apart, they might both be ideal airport locations.
- How will economic and sociological growth impact these equations? How should the ministry expect these proportions to change over the next decade? Over the next 30 years? 50 years?
India’s aviation officials wondered if it would be possible to find the optimal locations for their next set of airports by looking at search queries generated by India's internet-savvy citizens. In other words, could general digital activity help the Ministry understand factors like regional travel intent and consumer sophistication?
A new use for data: location-casting
The Ministry of Aviation partnered with Google to find the answer. For questions about customer intent and ability to pay, travel-specific searches like "how to get from Surat to Bengaluru" were an obvious place to start. Engineers parsed billions of accumulated queries, breaking them up into different combinations of origin and destination and looking at the frequency of travel intent among people in various regions in India.
But they also considered queries that were unrelated to travel. For instance, to find the first piece of the puzzle — areas with the greatest demand and affluence for travel — they knew that news-related searches and searches that indicate diversified economic activity tend to be most common in relatively developed areas. For example, freight forwarders are only present where economic activity demands it, similar to railways and airports. Searches for very specialized industries such as design schools or cancer research laboratories are also indicators of relative market readiness.
The search for "optimality"
The second piece of the puzzle is what Google has coined "optimality." Simply, each additional airport changes the demand pressure on the entire existing network. The optimal solution is one that serves the most people while reducing the most overhead for travelers (taking into consideration factors such as time and costs of travelling to and from the airport).
Evaluating India’s airport access using travel overhead
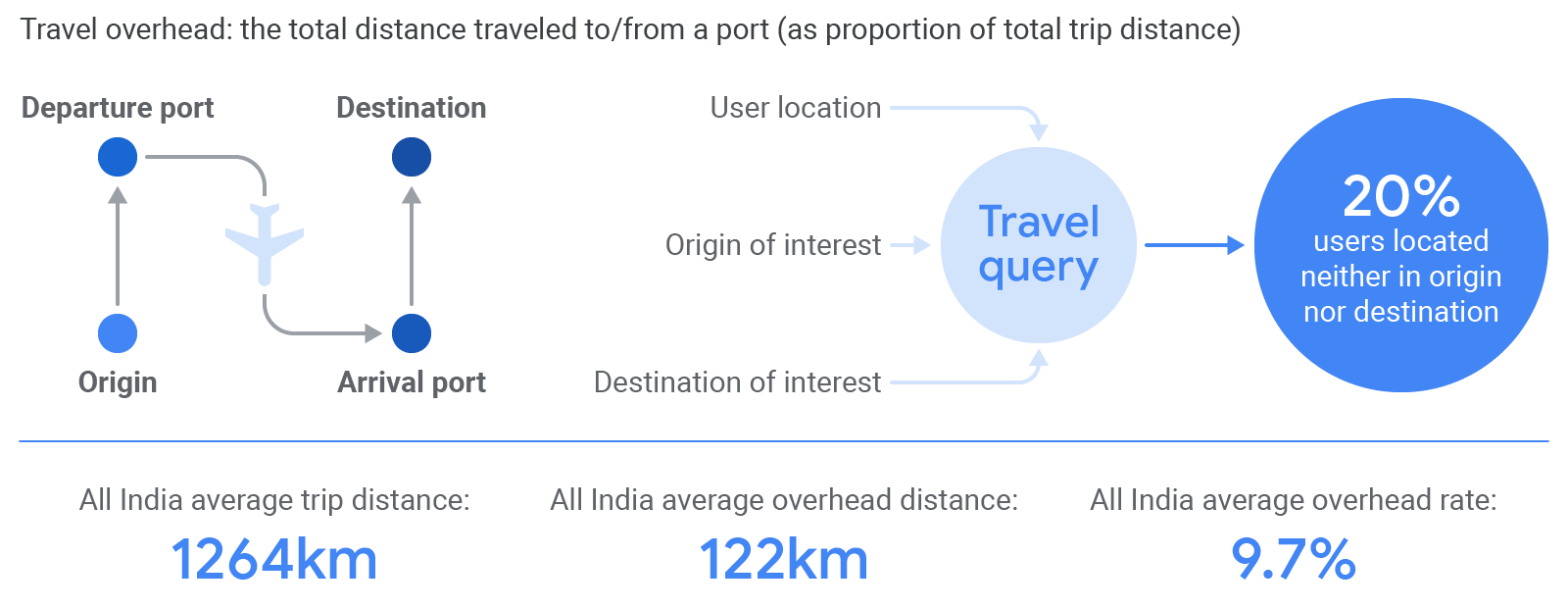
Google estimated that there were 75 billion possible ways to choose ten locations from 60 potential cities. With the help of machine learning, Google built 100,000 models using around 300 possible factors (such as public utilities, educational establishments, arrival and departure queries, etc.) and then ran 10,000 simulations of airport deployment to calculate maximum optimality.
The result? An intricate map showing how best to unlock India's latent flight demands. Google recommended ten "optimal" airport locations across India, ranging from Ludhiana (with a large population of 2.1 million) down to Mavelikara (with a small population of 300,000 but a large regional catchment). Google was also able to classify likely demand by type of travel, from tourist hubs (Puducherry) to educational travel destinations (Kharagpur).
The ministry eventually chose seven of the ten airport sites, and in the process of planning a subsequent round of construction, they chose another 30 from a list of 40 additional recommended sites.
A growing opportunity for brands
When it comes to location-casting, airport locations, while highly complex, are just the beginning. Location-casting offers a massive competitive edge for brands in almost any industry, whether they're building new retail outlets or deciding how warehouses should adjust their stock to meet hyper-local intent.
In today’s data-driven world, solutions like these are empowering business leaders to think beyond the norm and make data-informed business decisions.
To learn more about location-casting, download the white paper here.